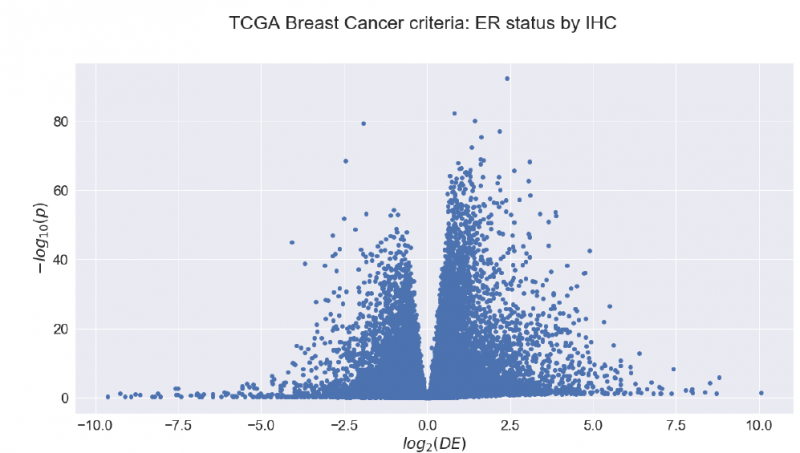
Connecting the dots: Systems Biology course review
The first semester of the second year of the Molecular Techniques in Life Science Programme (MTLS) has just come to an end, so its time for a course review! In this post I am going to describe my experience in the Systems Biology course, one of the electives taught by KTH.
My personal view on the course
What I liked most about the Systems Biology course is that I took a lot out of it. Most of the topics covered in class where completely new to me, and I got familiarised with trending topics in research such as combining different types of -omics data, or modeling metabolism. Specially, we had a lot of hands-on practice in the computer labs which helped me to understand the lectures.
However, the course is very intense, especially if combined with the Project in Molecular Life Science elective. I had to work very hard to keep up as good as possible with both courses, and that was definitely a challenge 🙂 Now looking back at it I am very happy that, even with the struggles, I took the course because I learnt a lot and I (hopefully) will benefit a lot from it in the future.
Lecture methodology: mixing traditional lectures and flipped-classroom.
The lectures involved two types of teaching methodologies: traditional lectures and flipped-classrooms. The flipped-classroom, which was used for the first three blocks of the course, consisted in providing us a series of readings and video lectures that we had to understand before the lecture started.
Moreover, before every of these flipped-classroom lectures we also had two assignments. One of them consisted in describing three concepts that we had learned while preparing the materials. The other was writing a question or doubt about the lecture topics.
The lecture would then consist on the professor going through the questions submited by the students.
What did we learn in the lectures?
The course covered very different topics in systems biology research, including statistics, metabolic models and integration of -omics data.
Block 1: Statistics
The course started with a “crash course” in statistics, focused on biological big data. For some people these were the hardest lectures to understand, but also the most useful ones because statistics is everywhere!
Block 2: Machine learning
The second part of the course focused on both supervised and unsupervised machine learning. I felt that this part was easier to understand, since we already had some other courses covering machine learning.
We did learn how some classifiers are built and work and understood both clustering and ANOVA in more depth.
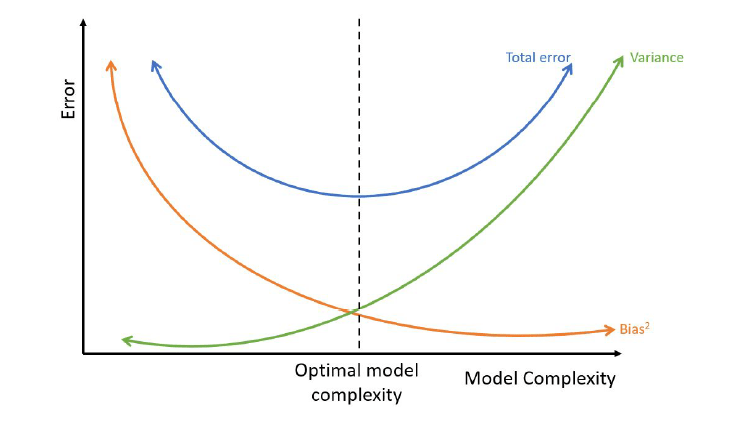
Block 3: Pathway and Network Analysis
There again, we already had some background in these topics, so these lectures were not the hardest. I am really interested in these topics, so I found them fun! They are also applicable to every day´s work at research labs, and it is good to know the basics of it even if you are not working on it.
Block 4: Genome-Scale Metabolic Models
This topic was brand new for me, and I have to say I liked it! We learned how metabolic networks are modeled mathematically, and how can they be used to predict the growth of bacteria in certain media, or to find genes that are essential for the survival of an organism. Genome-Scale Metabolic Models are abundantly used in both academia and industry, so this part of the course satisfied the interest of both types of students.
Block 5: Integration of different -omic data types
The last block of the course was actually very helpful to me. I had heard many times that different data types can be merged together, but the reality of it is always more difficult than it seems. The last part of the course gave some insights into it, as well as how boolean gene regulatory networks are built and interpreted.
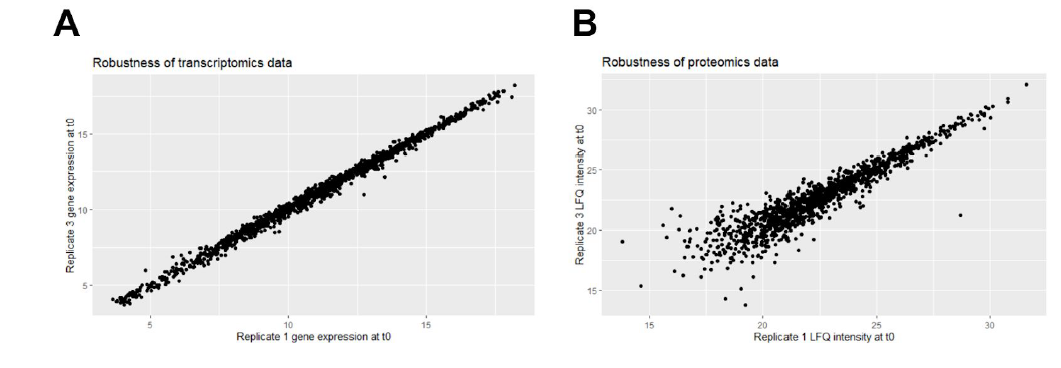
Computer Labs – Putting our skills into practice
To practice what we had learned in the lectures, we had to do four computer labs distribute as follows:
- Statistics
- Machine learning and pathway analysis
- Genome-Scale Metabolic Models
- Integration of proteomics and transcriptomics data
All the labs were done in pairs and had two parts. The first part was the basic, mandatory part that had to be successfully completed to pass the course. Each lab had different exercise types, but all the mandatory parts made sure to cover all basic concepts explained in the lectures. The second part of the labs was a bonus part, that was voluntary and would give “extra points” on the exam if completed successfully. Here again, the requisites for each lab varied a lot, but the bonus questions were generally more advanced and required more programming.
I did the computer labs with my classmate Kavan, and they were very, very useful to understand the course and exam materials. We had two weeks to complete each lab (both basic and bonus part), and it did happen that almost all groups had to squeeze in a lot of work hours right before the deadline, but we were much better prepared for the exam after completing them.
I also want to mention the hard work that all teaching assistants did during these computer labs. We were always welcome to ask as many questions as we wanted, and they did their best on answering them.
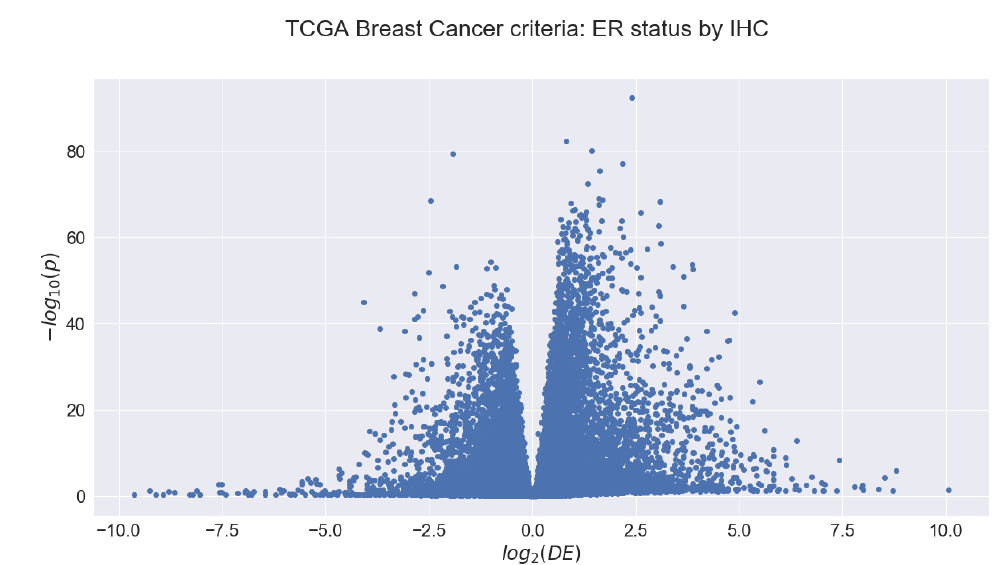
I hope this course review was useful for you out there who are thinking about applying for the Molecular Techniques in Life Science Joint Master Programme, or for you current MTLS students that need to choose the elective courses next semester.
See you next time, and do email me if you have any questions about the MTLS Programme or life in Stockholm 🙂
\Inés
email: ines.rivero.garcia@stud.ki.se
LinkedIn: Inés Rivero García
0 comments